Gradient Accumulation
Gradient accumulation (GA) enables reduced GPU memory consumption through dividing a batch into smaller reduced batches, and performing gradient computation either in a distributing setting across multiple GPUs or sequentially on the same GPU. When the full batch is processed, the gradients are the accumulated to produce the full batch gradient.
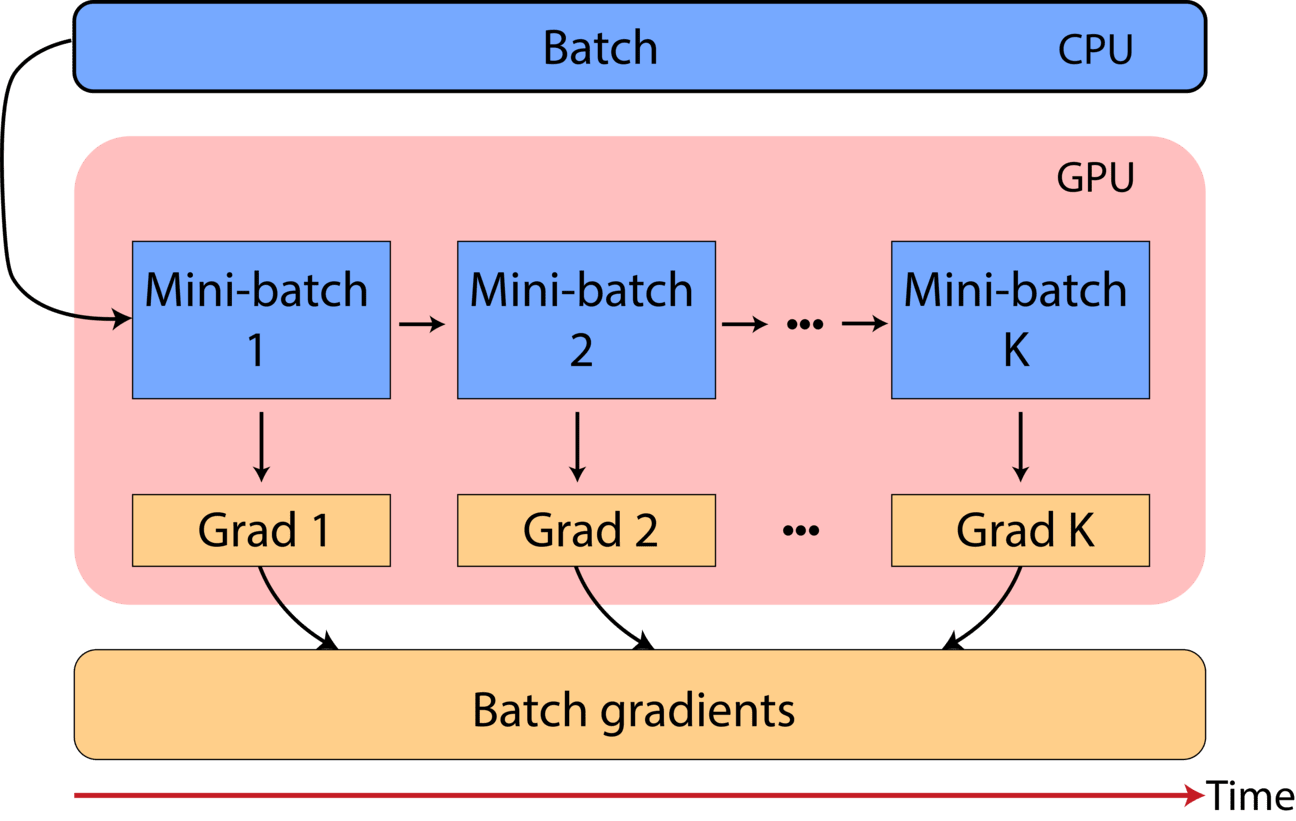
Gradients for K mini-batches of size M are calculated, before being scaled by 1/K and summed. After K accumulation steps, the overall gradient is produced and the weights are updated. By doing so we approximate batch training of K * M, without the need to keep the entire batch in memory.
A simple usage example can be seen below:
from tensorflow.keras import Model
from gradient_accumulator import GradientAccumulateModel
model = Model()
model = GradientAccumulateModel(accum_steps=K, inputs=model.input, outputs=model.output)
model.compile(optimizer="adam", loss="cross-entropy")
model.fit(train_set, epochs=10, batch_size=M)